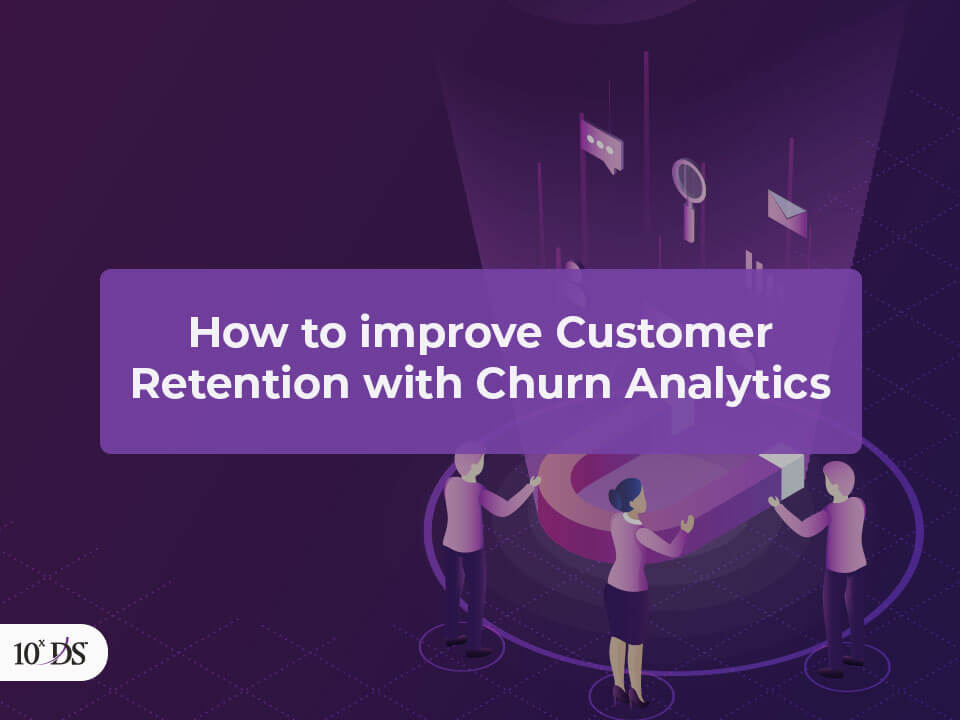
How to improve Customer Retention with Churn Analytics
For any company, winning a new customer is a long-drawn process involving a lot of hard work and struggle. At the same time maintaining and nurturing long-established clients definitely has its own set of unique challenges. According to Invespcro, it costs five times as much to attract a new customer than to keep an existing one. A business would benefit from predicting and understanding customer churn, removing some of the guesswork out of customer retention probability. Now, Customer Churn means the rate at which customer leaves your company, organization or service also called the rate of customer attrition. Analysing behaviours of the clients who have churned in the past and closely observing the current customers behaviour for similarity, companies can proactively take actions to positively affect their retention. According to Bain & Company, increasing customer retention rates by 5% increases profits anywhere from 25% to 95%. Today, it’s more about being creative in the targeted plans offering customers something as personalized as possible, so that they feel truly special, nurturing loyalty in every one of the customers.
Understanding customer churn analytics
It is extremely important for companies of this day and age to have a strong customer retention strategy to reap rewards, if you do it right, to drive profit. The success rate of selling to a customer you already have is 60-70%, while the success rate of selling to a new customer is 5-20%, according to Invespcro. Companies across the globe have started thinking of the possibilities of the use of analytics, but they do not seem to leverage it enough, or customer churn analytics, to its full untapped potential to create a competitive advantage. According to Forester, in its B2B Marketers Guide to Benchmarking Customer Maturity (2017), only 15% of senior leaders actually use customer data consistently to inform business decisions.
When we compare companies, customer churn is a major challenge especially for B2C companies. If we take the example of the telecom companies, their target market is huge and the consumers have several easy alternatives to choose from, and more importantly there is not much difference in the offerings compared to the competitors. Leveraging traditional analytics companies can analyze how many customers are leaving customer base and the profile of customers that are leaving and entering the customer base. Analyzing this data would help companies go a level or two deeper and slice the data based on various attributes and dimensions to understand what is causing the churn. However, despite all the key data points available using the data visualization tools, the user still needs to understand how all customer attributes work in concert to influence churn and comprehending it is difficult because human brain has its limitations analysing multiple dimensions at the same time.
Ways to reduce churn using churn analytics
1. Define roadmap to implementing analytics solutions
Companies leveraging data and analytics need to implement the organizational changes based on the insights gleaned from the data. The process is always better if there is a definite roadmap and steps such as defined KPIs and problems, categorizing data and issues, prioritization of tasks with preparation of technical feasibility, tracking progress and ensuring behavioural change in the staff to use customer analytics. It is important to develop the necessary skills in the team to analyse the data. Senior leaders should be an example leading the employees to stay committed to using data analytics, and internal teams should communicate with each other.
2. Analysing potential customers
If companies have more comprehensive data about their customer behaviours and understanding of the reasons for the churn, they can target customers that are less likely to churn, by setting the loyal customers as a benchmark. Some of the parameters or characteristics that can be assessed is the employee count, annual spend, type of industry, job titles etc. compared to existing customers. This helps companies to filter and target those companies that are likely to look for service or products of the company, find it useful and therefore stay loyal.
3. Leveraging Predictive models and machine learning
Predictive analytics can help companies make predictions, by leveraging historical data of the customers to check their preferences. Machine learning can make things easy for data analysts to quickly and accurately uncover the real reasons why customers leave or why some are loyal to your brand. Machine learning can help to get ahead of customer churn seeking patterns in the data. The machine learning models applied can provide more details about the customer attributes. The models can be used to create customer profile groupings and associations, to know which customers are most likely to churn and who are the most loyal customers. This is a very useful information to take actions on product and package development, for introducing features catering to right audiences. Marketing also becomes far more easy allowing companies to target the right audience with appropriate messaging.
Benefits to companies using Churn Analytics
One of the top benefits for companies using churn analytics is that selling to existing customers becomes easier and cost effective. Churn analytics helps you identify factors leading to customer churn and companies can proactively launch targeted campaigns and strategies to abate customer attrition. Another important benefit is to create strategies for re-acquisition of the lost customers. Finally, averting customer loss in the first place by analysing the churn indicators and this can significantly help companies in everything from revenue to opportunity for competition.
Use Case: Reducing customer churn using Predictive Analytics
A telecom company was facing the challenge of high churn rate, having to spend significantly to acquire a new customer. The company loses the opportunity of possible revenue from customer but also the money, time and resources spent to acquire the customer. A predictive churn model was developed using data such as complaints data , billing, delivery, assurance, and customer satisfaction to identify key factors leading to the churn. Using techniques such as regression analysis several models were built for each business units and service, all adding up to create a churn model. The solution enabled advanced reports and dashboards to predict customers leaving their service. The insights from the report helped the management team make right business decisions to prevent customer attrition. The insights can help the management to create a sustainable and robust strategy for customer retention and formulate ways to reacquire the lost customers. Customer satisfaction levels can be tracked by product, segment, cost to serve and others.
How 10xDS can help?
10xDS is driving digital transformation by leveraging our expertise in data and analytics technologies and deep sector experience to enable organizations to make effective strategic decisions. Our strong portfolio of data and analytics services include Information Management, Big Data, Business Intelligence and Advanced Analytics solutions to help assisted and semi-automated decision making.
Interested in learning more about customer churn analytics or implementing the solution in your organization, but not sure how to proceed? Talk to our Data and Analytics Experts!