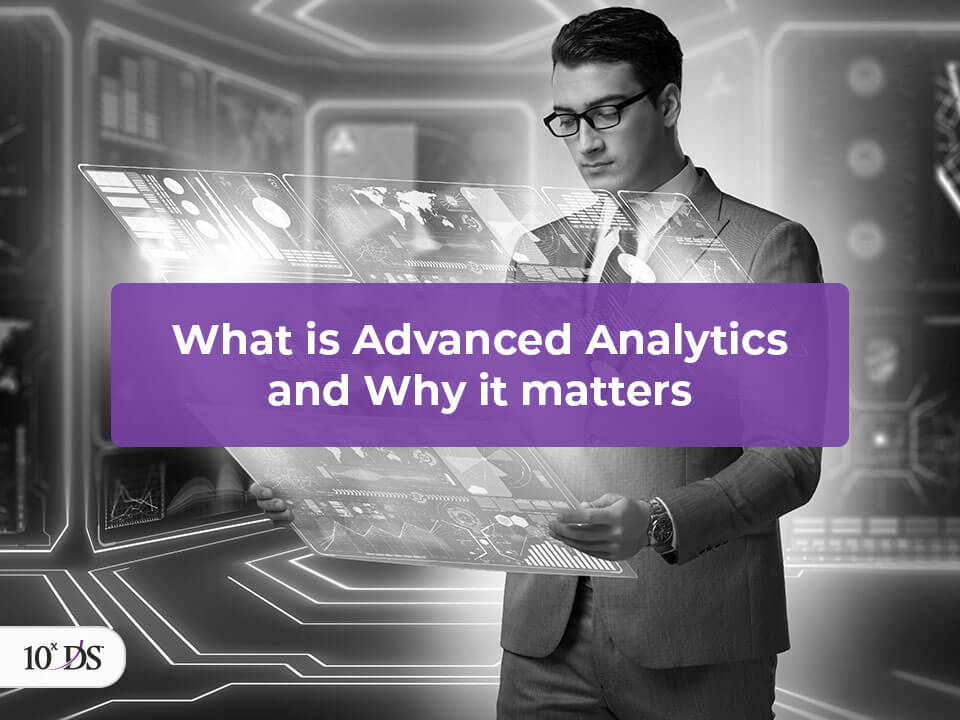
What is Advanced Analytics and Why it matters
Advanced analytics is a part of data science leveraging advanced complicated methods and techniques designed to parse, explore and analyze data and produce results to support business decisions. In the last decade, we have witnessed some dramatic changes in the technology world and big data and the new emerging data analysis techniques are at the forefront of this revolution. We might have heard about Advanced analytics and its tremendous potential to create value, but let’s understand “What is Advanced Analytics?”.
Advanced analytics is not just one particular model of technology, its actually a collection of various powerful techniques used to gain actionable insights for better decision making. Using Advanced Analytics, organizations are empowered by the ability to perform advanced statistical models and streamline various aspects of their operations. It is different from traditional Business Intelligence (BI) where data is processed to gain insights on the past performance of business operations. Advanced Analytics helps solve complex business problems. Starting from small, advanced analytics leverages statistical methods and enhanced computational power to capture specific characteristics and habits of individual customers, going beyond the analysis of customer segments. This can create targeted and personalized marketing campaigns and improve marketing ROI.
Advanced analytics has four major branches: Data mining, Predictive Analytics, Prescriptive Analytics and Big data analytics. Let’s have a look at each branch and techniques and understand why it matters to companies:
1. Data Mining
Data mining is an advanced analytical method used for sifting through and extracting usable information from large datasets. It is used to uncover patterns, trends, and other hidden insights. Data mining is performed leveraging machine learning and statistics. Some of the most common techniques include Tracking patterns in large datasets is one the common techniques of data mining, recognizing some aberration in the data happening at certain intervals. For example, you might see that your sales of a particular line of product see a significant increase just before the holiday season. Clustering – It involves grouping large datasets together based on similarities within the data. For instance, you might choose to cluster or segment different demographics of your audience into different incomes they have, or their shopping track record. Regression Analysis is another commonly used technique to analyse the effect of one variable on many others. One popular example is how Body Mass Index (BMI) affects other aspects of health.
2. Predictive Analytics
Predictive analytics is an emerging branch of advanced analytics which uses techniques such as statistics, modelling, machine learning, Artificial Intelligence and others to provide best assessment of what will happen in the future. Many organizations are turning to predictive analytics for cracking difficult and challenging problems to uncover untapped opportunities. Some of the common uses of Predictive analytics include marketing campaigns optimized to determine possible customer responses, identifying cross-selling possibilities, attracting new businesses and retaining customers. Another very popular use is to reduce risk by assessing the likelihood of loan default. Fraud detection is increasingly becoming a common use of predictive analytics. Leveraging multiple analytics methods, organizations can improve pattern detection, prevent attacks, spot abnormalities that may indicate fraud and persistent threats.
Financial institutions spend a lot of money and effort on the maintenance of ATMs.Organizations could save money by reducing such operational costs if they had insights into which ATMs are nearing maintenance, thus enabling them on better utilization of the maintenance staff and reducing operational costs. In this case, advanced analytics and machine learning techniques can be used to predict the probability of ATM failure. To enable this, past data needs to be consolidated from past failure logs and other sensor readings of various components of the ATMs. Predictive models could be utilized on regular intervals to get the probability of the failure of the various ATMs based on the recent data collected from these machines.
3. Prescriptive Analytics
Analytics can be classified into three distinct branches segments – Descriptive, Predictive and Prescriptive Analytics. In descriptive analytics historical data is analysed to identify patterns and trends. Predictive Analytics go the next level as it answers the question as to what is likely to happen. Finally, Prescriptive analytics, the last stage of analytics where the predictions are used to prescribe the next set of things, recommend actions you should take to optimize a process, campaign, or service to the highest degree. Prescriptive analytics gathers data from a variety of both descriptive and predictive sources and combines existing conditions and possible decisions to determine the impact of a decision based on different possible future scenarios. Most modern analytics tools have prescriptive analytics already built in providing companies actionable results empowering them to make better decisions. Interesting example is in the oil and gas sector where prices keep fluctuating based on ever-changing political, environmental, and market demand conditions
4. Big Data Analytics
Big data analytics examines large digital stores of information that have a high volume, velocity and variety to uncover hidden patterns, correlations and insights. Several companies are implementing Big Data Analytics for making more informed business decisions. Data scientists, predictive modelers and other analytics professionals can now analyse Big Data from multiple and varied sources, including transactional data and other structured data. There are various types of tools that can be grouped under the umbrella of Big Data Analytics such as data storage and management, data cleaning, data mining, data analysis, data visualization, data integration, and data collection.
Big data analytics is useful in Real-time forecasting and monitoring of occasions that may affect the performance or operations of the businesses. It helps mitigate risks by optimizing complex decisions about unplanned events faster and better. Companies can gain better understanding of the potential of data-driven marketing. Companies can improve customer satisfaction by customization of customer experience, adding value to interactions with online and offline customers and increasing loyalty in the long run.
Bigdata technologies could also help in building an efficient screening process, which processes massive data volumes including inhouse, social media and publicly available information about the customer.
Conclusion
Analytics solutions are quickly transforming the banking world, improving profitability, compliance, competitiveness and helping shape business decisions. Developing Advanced Analytics capabilities may seem daunting at first, but you can start with small doable steps, integrating data analytics into operating models and expand from there to stay ahead of the competition. To learn more about how to kickstart your analytics initiative and efforts to enable smarter decision-making. Talk to our experts!