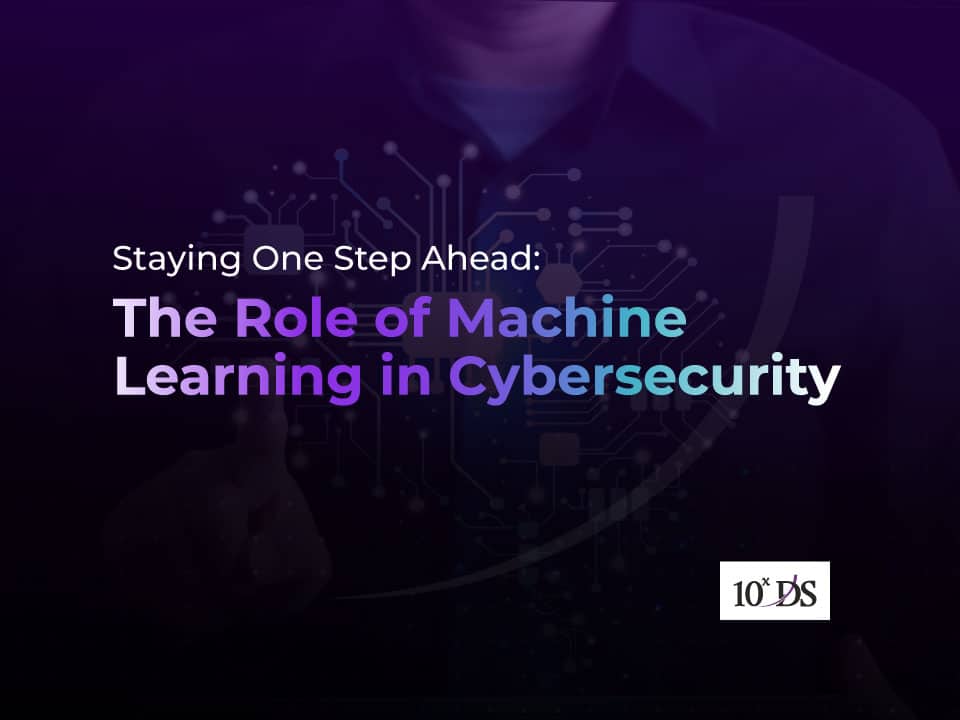
Staying One Step Ahead: The Role of Machine Learning in Cybersecurity
Machine learning, an innovative branch of artificial intelligence, has rapidly transformed industries and revolutionized the way we interact with technology. With its ability to empower machines to learn from data and improve their performance without explicit programming, machine learning has opened new horizons across diverse domains. The growth of machine learning is astonishing, as evidenced by a staggering increase in its adoption and investment. According to a report by Grand View Research, the global machine learning market size was valued at USD 8.43 billion in 2020 and is expected to reach USD 117.19 billion by 2028, with a CAGR of 39.2%. This meteoric rise is further echoed by Sundar Pichai, CEO of Google, who stated, “Machine learning is a core, transformative way by which we’re rethinking how we’re doing everything.” Indeed, as machine learning continues to progress, its potential to drive innovation, optimize processes, and tackle complex challenges seems boundless. AI, and specifically machine learning, has emerged as a game-changer in the field of cybersecurity. As cyber threats become increasingly sophisticated and prevalent, traditional security measures often fall short in detecting and mitigating these risks. AI’s ability to analyse vast amounts of data, identify patterns, and learn from past incidents has significantly enhanced cybersecurity defenses. Machine learning algorithms excel in detecting anomalies, identifying potential malicious activities, and predicting emerging threats. They bolster early detection, enhance incident response, and empower security teams to proactively safeguard against evolving attacks. By leveraging AI and machine learning, organizations can stay one step ahead of cyber adversaries, fortifying their defences and preserving the confidentiality, integrity, and availability of their critical assets.
The evolution of machine learning has significantly impacted the field of cybersecurity, enhancing the ability to detect and mitigate cyber threats. Here’s how machine learning evolved to enhance cybersecurity:
Early Detection Systems
In the early stages, machine learning was used to create intrusion detection systems (IDS) and intrusion prevention systems (IPS). These systems analysed network traffic patterns and identified anomalies that might indicate potential cyber-attacks. Traditional rule-based systems struggled to keep up with the rapidly evolving threat landscape, but machine learning algorithms brought a dynamic and adaptable approach to identifying new attack patterns. Machine learning leverages its ability to analyse vast and diverse datasets, such as network traffic, user behaviour, and system logs, to identify anomalies and potential threats. By learning from historical data, machine learning algorithms can establish baseline patterns and detect deviations in real-time, signalling the presence of emerging cyber-attacks or intrusions. These adaptive systems continuously update their knowledge, enabling them to stay ahead of evolving threats and providing organizations with the opportunity to proactively respond and defend against potential breaches before they escalate into more significant security incidents.
Behaviour Analysis
As cyber threats became more sophisticated, signature-based approaches were no longer sufficient. Machine learning algorithms enabled the analysis of user and system behaviours to detect deviations from normal patterns. By understanding typical user behaviour, anomalies such as unusual login locations, atypical data access, or suspicious network activity could be detected, helping to identify potential insider threats and advanced persistent threats (APTs).
Malware Detection
Machine learning has significantly improved malware detection by leveraging features from known malware samples to identify new and previously unseen threats. Machine learning models can learn to recognize patterns in malware code, behaviours, and network communications, allowing them to detect and categorize malicious software more accurately than traditional signature-based methods.
Phishing and Spam Detection
Phishing attacks and spam emails continue to be significant cybersecurity challenges. Machine learning models can analyse the content, sender characteristics, and context of emails to identify potential phishing attempts and spam messages. This helps in preventing users from falling victim to social engineering attacks.
User Authentication
Machine learning techniques have also been utilized to enhance user authentication and access control. By analysing historical user behaviour and contextual information, adaptive authentication systems can determine the risk associated with login attempts and apply appropriate security measures, such as multi-factor authentication, when suspicious activities are detected.
Predictive Analysis and Threat Intelligence
Machine learning models are employed to analyse vast amounts of data from various sources to provide predictive insights on potential cyber threats. By analysing historical data and patterns, these models can predict future attacks and vulnerabilities, helping organizations proactively strengthen their cybersecurity measures.
Network Security
Machine learning plays a crucial role in improving network security. It aids in anomaly detection in real-time, detects distributed denial-of-service (DDoS) attacks, and identifies potential network intrusions.
One early adopter of machine learning in cybersecurity is Darktrace, a leading AI cybersecurity company. Darktrace’s “Enterprise Immune System” uses unsupervised machine learning to create an evolving baseline of normal behaviour within an organization’s network. By continuously monitoring network activity, Darktrace’s AI system can quickly detect deviations from the norm, indicating potential threats such as insider threats, zero-day attacks, and advanced persistent threats. This early detection and proactive response allow organizations to neutralize threats before they escalate, thereby strengthening their overall security posture. Similarly, Palo Alto Networks, a cybersecurity leader, utilizes machine learning in their “WildFire” service. This cloud-based platform analyses suspicious files and URLs using advanced machine learning models to identify and prevent previously unknown threats. Symantec’s “Endpoint Protection” solution also utilizes machine learning algorithms to analyse file behaviours and identify potential threats in real-time, enhancing their threat detection capabilities. These are just a few examples of how machine learning is being harnessed by cybersecurity companies to improve threat detection, enhance incident response, and strengthen overall security defenses. As the technology continues to advance, more organizations are likely to adopt machine learning in their cybersecurity strategies to stay ahead of evolving threats.
Talk to our experts to know more.