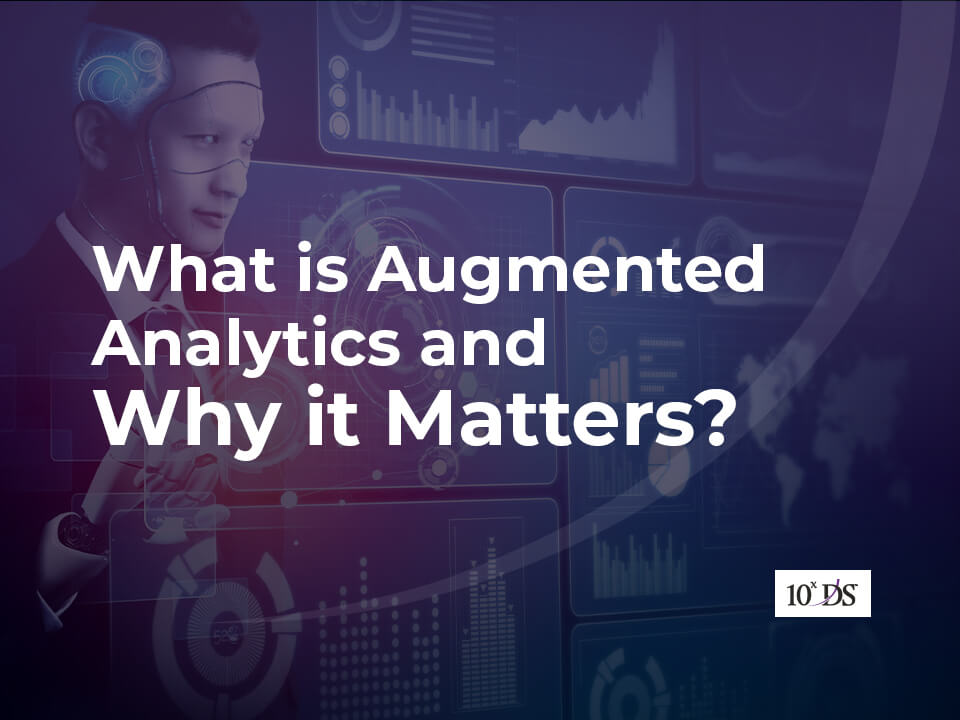
What is Augmented Analytics and Why it Matters?
Augmented Analytics has been one of the most talked-about technologies of late that refers to using Artificial Intelligence (AI) and Machine Learning (MI) to make the most of data. Gartner explains Augmented Analytics as a potential tool that can help with “data preparation, insight generation, and insight explanation to augment how people explore and analyze data in analytics and BI platforms”.
The global research giant further states that Augmented Analytics can improve the way data scientists manage their day-to-day jobs by helping them to automate “many aspects of data science, machine learning, and AI model development, management, and deployment”. Augmented Analytics can also help to enhance business intelligence in a variety of ways by facilitating automation, data discovery, conversational analytics, and generating contextual insight suggestions.
Core Benefits of Augmented Analytics
Augmented Analytics can process data much faster than humans could while remaining unbiased at all times. In a traditional business intelligence model, analysts use their theories and hypotheses to evaluate data, which means that they are relying on a specific range of knowledge. This can limit their viewpoints beyond the areas they are adept in, which can create many challenges to derive a comprehensive and unbiased conclusion. Moreover, the accuracy of the data analysis can also fluctuate since the conclusion is made without assessing every possible factor.
These challenges can lead to major investment risks, as businesses will be potentially functioning with limited insights on their data landscapes. With Augmented Analytics, data processing and analysis can be made more efficient and accurate by tapping into the power of ML and AI. When coupled with Natural-Language Generation (NLG), it can generate the most comprehensive outlook on the available data. Meanwhile, Augmented Analytics can also break it down to simpler terms so that businesses can understand it and take relevant actions to drive better productivity.
Here are some of the key advantages of Augmented Analytics, which can help enterprises to make improved decisions for their business growth.
1. It Delivers More Value
One of the clear perks of combining data science and AI is that it leads to faster data preparation and speedy visualization. Users can also gain accelerated insights on the data at hand, which translates to higher productivity. Besides, Augmented Analytics can also replace manual processes using data preparation algorithms, which will free up data scientists to work on more complex tasks.
2. It Opens Up More Possibilities
While typical business intelligence solutions need a hypothesis or idea to work, Augmented Analytics can uncover hidden insights using AI algorithms and contextual suggestions that data analysts could not have spotted otherwise. In turn, users can gain more confidence in finding further insights using data science and AI tools when they discover hidden relationships, correlations, and outliers in data.
3. It Helps to Increase Reliability
Whenever users interact with data on the system, they inform ML algorithms about the business context, intent, and skill set. These algorithms collect this information and learn from it over time to provide more relevant and accurate suggestions. This boosts trust and interactions in the analytical process, which leads to buy-ins and wider adoption of analytical solutions in the organization.
Apart from these, Augmented Analytics can also improve data literacy in due course as it collects massive amounts of data. This will allow analysts to make recommendations based on automatically surfacing insights, and confidently take the necessary action based on the results.
Drawbacks of Augmented Analytics
Although Augmented Analytics can help with faster results by automating routine tasks concerning data preparation, analysis, and visualization, it is not devoid of some challenges either. Enterprises using data science and AI solutions will always need to ensure that the insights generated are accurate and reliable. This means working with comprehensive datasets that are free of errors while upgrading the existing models as per the data change.
Relevancy is another concern, as users do not usually filter out unrelated information. This means that data scientists will need to test the data to ensure its relevancy before it is processed by Augmented Analytics tools so that it produces the most accurate results. Likewise, organizations will need to train the Augmented Analytics model with enough data at first to start gaining valuable insights.
Augmented Analytics can also consume a lot of computing power and resources depending upon the platform and its capabilities. This could lead to a slow response time when processing large volumes of data. On the other hand, upgrading the computing resources to use data science and AI models can lead to more capital investments, which may not be a practical solution for some businesses.
Conclusion
The best way to make the most of Augmented Analytics is to start small and see how the results benefit the business. Choosing a use case that aligns well with the KPIs of the company and has higher business value can work well to measure the success of the combined data science and AI model. Similarly, it is advised to train and educate analysts to become a part of the Augmented Analytics system so that there can be better transparency in generating insights.
Talk to our experts to learn more about Augmented Analytics and see how it can help to build a data-driven culture across your organization. By ensuring that your analysts become familiar with using the technology, you can definitely get the most out of your business’ valuable data assets.