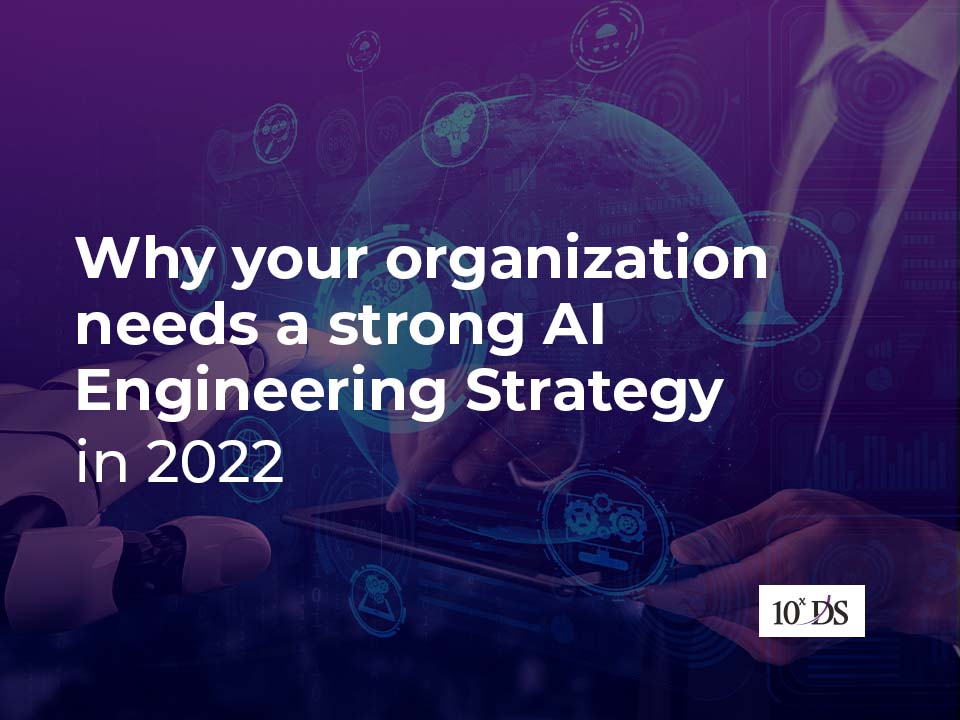
Why your organization needs a strong AI Engineering Strategy in 2022
Artificial Intelligence (AI) engineering is one of the topmost trends in Gartner’s Top Strategic Technology Trends. AI technology is driving advances throughout the globe in various sectors such as education, BFSI, government sector, medicine, transportation, and more. Though in the current landscape, AI technologies and systems are being rapidly developed, the technology and discipline of AI engineering is in its nascent stages and evolving. Products and services with AI capabilities enabled can lead to significant benefits for enterprises. AI adoption offers increased efficiency, automation of manual processes, better decision making, enhanced customer service, among others.
However, according to the research firm Gartner, several organizations find it challenging to realistically evaluate the impact of AI. Many companies tend to overestimate the impact of the technology and underestimate the overall complexity. Garter published a report on AI in organizations in 2020, based on a survey of more than 600 senior-level executives from several enterprises in countries such as the U.S., Germany, and the U.K. The report says that one third of the enterprises have already deployed AI, over half of the organizations surveyed planned to deploy AI in less than one year, and nearly one third planned to deploy the technology within two years. The report also says, though there is a huge shortage of skilled workers in AI, that’s not the main factor affecting the successful deployment of AI solutions. The major challenge is the lack of clear outcomes. This is where an engineering approach to AI can be useful for organizations
Overview of AI Engineering Approach
AI engineering addresses the challenges of AI projects in aspects such as maintainability, scalability, and governance. According to Gartner, following a sound and robust AI engineering approach will enable better performance, scalability, interpretability, and reliability of AI models while ensuring greater ROI from AI investments. AI engineering brings the technology under the mainstream DevOps process. Combining various disciplines, AI engineering provides greater value by operationalizing multiple AI techniques put together. AI engineering would require capabilities such as extracting data from multiple sources, algorithm designing, building and testing machine learning (ML) models, and then implementing those models to build applications capable of performing tasks of varying complexities. AI engineering gives high importance to governance, considering aspects such as trust, transparency, ethics, fairness, interpretability, and compliance issues in AI projects
AI Engineering Strategy
AI projects usually have two types of cycles. Depending on the nature of its application, the first cycle involves development and training of ML models, for instance, rule-based models, optimization models, or natural language processing models. The second cycle focuses on the implementation of these models within an enterprise. As far as strategy goes, many organizations have grown to be adept at developing models, but where they fail is in the production of these models. AI engineering helps in systematizing the production of these models.
AI will grow and mature in the future, aligning with the rapid innovations happening in the tech space. Companies leveraging AI engineering will create and maintain business solutions that incorporate AI models and techniques. Organizations should ensure that the system requirements are properly mapped and driven by business needs and suitable AI techniques and strategies are selected for those requirements. Moreover, there should be proper testing, monitoring process and tools in place for the smooth functioning of the system.
Key components of AI Engineering
1. Understanding the use of AI in the business
If an organization needs to successfully tap into the many benefits of the use of AI, it’s important to understand that moving to the operational phase is not just another technical aspect, but a business one as well. When an organization puts AI to production, it is necessary to speak to subject matter experts in properly understanding the applications they are running, use cases for AI and how these applications help and impact the business. Organizations need to place the business users at the heart of their AI engineering practice to ensure the proper delivery of AI initiatives.
2. Quality of data
Another key aspect that needs to be considered is the quality of the data that companies will feed into models. It’s important to understand where the data is coming from and how reliable it is. DataOps, a new and evolving discipline, can help enterprises improve data quality. DataOps is an automated and process-oriented methodology leveraged by data analytics teams to enhance the data quality and bring down the cycle time for analytics. DataOps encompasses the complete data lifecycle, from data preparation to reporting, and the methodology considers the importance of the data analytics and IT operations teams working together.
3. AI Skillset
Another key component for organizations looking to use AI is to ensure that the right skills are in place. For instance, organizations should have people with experience in programming languages such as Python, R, Java, C++, and others. Other necessary skills include capabilities in machine learning, data science, neural network architectures, understanding of applied mathematics including probability and statistics, and also experience in the latest technologies such as robotics and computer vision. Organizations should have proper upskilling and education practices to acquire these skills internally. They can also look to hire new talents to maintain the right talent mix needed to be successful.
Conclusion
Several organizations have realized the importance of AI in providing real business value and they have started building up their data science, machine learning, and AI teams. Organizations should have a holistic approach to realizing, operating and maintaining AI for their business. They should have proper AI engineering strategy in place to properly resolve the AI issues and improve the chances of the AI models to find their way to production. A structured engineering approach to AI can fix the AI issues faced when moving from the development and training phase to the operational/product phase. Organizations that have systematically implemented AI are now reaping the many benefits out of it.
Talk to our experts to better understand the AI engineering approach and help you choose the right AI technology adoption for your organization to streamline your business processes and drive better value.