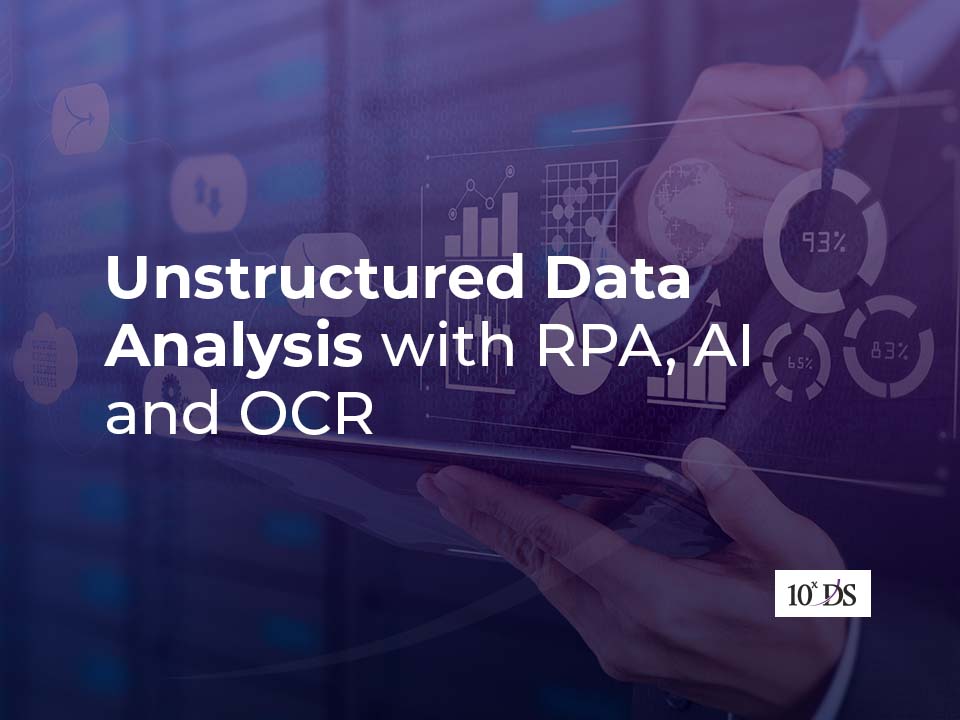
Unstructured Data Analysis with RPA, AI, and OCR
RPA has been helping businesses automate processes with structured data but unstructured data which constitutes 80 to 85% of all enterprise data cannot be automated using only traditional RPA tools. Capturing, classifying, extracting, enriching, and processing unstructured data such as emails, contracts, handwritten documents, PDF documents and scanned documents requires judgement and decision making. Processing the data manually from these unstructured data sources is not a viable option due to the high operational costs, long turnaround time and high possibility of errors. The traditional rule-based or template-based approaches that involve large volumes are time-consuming to maintain.
Considering all these factors, enterprises are resorting to Artificial Intelligence (AI) based Intelligent Automation (IA) solutions like computer vision, machine learning (ML), natural language processing (NLP), deep learning, etc. These solutions can be integrated with RPA and OCR for end-to-end processing of unstructured data sources to be digitally competent. The AI based solutions are very effective in processing unstructured data in industries such as healthcare, banking and financial services thereby boosting business value and offering a competitive advantage.
Limitations of RPA and OCR in processing Unstructured Data
RPA together with OCR and template-based automation converts document images into machine-encoded text and extracts fields based on a specific template but they do not have the capability to process unstructured data and have the following limitations:
- Classification of documents into different categories is not possible
- Every document that is converted to machine-encoded text needs to be manually reviewed unless the input documents are standardized
- Cannot assist/help the user while reviewing the extracted information
- Cannot process unstructured documents such as contracts and emails
Due to the above-mentioned limitations, the role of AI is crucial to achieve end-to-end automation of unstructured data.
Role of AI and related technologies in processing Unstructured Data
AI technologies provide businesses with the capability to capture data from different channels and to build up RPA, OCR and other rule/template-based automation technologies. In addition, they can also provide insights from unstructured data sources with the help of deep learning, Machine learning, Transfer Learning or NLP capabilities. The AI solutions can use cognitive skills at each of the below steps of the content processing value chain for unstructured data described below:
1. Document Capture
In this stage, the underlying technologies Computer Vision and OCR are used for Document capture and recognition from sources such as tweets, emails, documents (including PDFs), scanned forms, handwritten documents, and images. The response is generated in natural language format using Sentimental analysis and Natural language generation features.
2. Document Classification
Once the document capture and recognition are completed, technologies such as Text Mining and Machine Learning are employed to do the document classification. The output format is in the form of a summary dashboard based on the extracted data and insights can be provided based on descriptive, predictive, and prescriptive analytical capabilities.
3. Data Extraction
Post completion of document classification, NLP, machine/deep learning is used to extract data in a structured output form and provide the data to downstream applications such as RPA, ERP and CRM systems using integration capabilities and pre-built connectors.
Key technologies in an Intelligent Automation solution for Processing Unstructured Data
1. OCR/ICR
OCR/ICR converts document images into machine-encoded text and is trained using ML and deep learning algorithms for improved accuracy.
2. Computer Vision
Computer Vision helps in the automatic extraction, analysis and understanding of useful information from digital images.
3. Machine Learning and Deep Learning Models
IA solutions use built-in ML and Deep Learning models are used to classify and extract documents, software training and image pre-processing to complement OCR.
4. Natural Language Processing (NLP)
NLP helps IA solutions to analyse running text in documents, understand the context, consolidate the extracted data, and map the extracted fields to a defined taxonomy. In addition, NLP is also used to recognize sentiments from text such as emails and classify them into different categories.
Conclusion
Enterprises have realized that they cannot process unstructured data using RPA alone and that they would need intelligent automation (IA) solutions like Computer vision, Machine Learning and NLP to provide end-to-end automation capabilities. Such IA solutions can help enterprises derive structure and meaning from unstructured data resulting in better business insights and smarter business decisions.
If you are looking for help to process your unstructured data, talk to our experts.