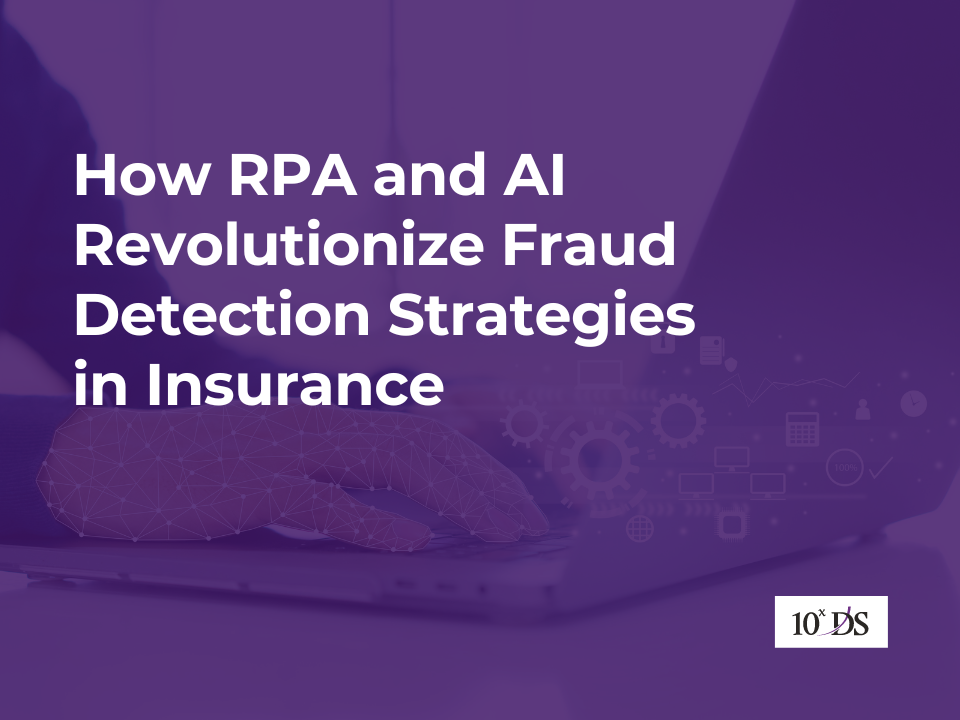
How RPA and AI Revolutionize Fraud Detection Strategies in Insurance
Fraud, a pervasive and complex phenomenon, transcends industries, leaving a trail of financial losses, disrupted operations, and compromised trust in its wake. Defined by deceptive practices aimed at gaining an unfair advantage, fraud manifests in various forms, challenging the foundations of businesses, institutions, and systems worldwide. Whether it be insurance, finance, or any sector vulnerable to deceit, understanding the dynamics of fraud is crucial for implementing robust countermeasures. This blog delves into the intricate facets of fraud in the Insurance sector, examining its impact, strategies devised to combat it using AI and Automation, and the ever-evolving landscape in which this age-old challenge persists.
Insurance fraud is unfortunately not a rare occurrence. There are several types of insurance fraud, including:
- False Claims: Individuals may submit false or exaggerated claims for losses or damages that did not actually occur in auto, property, or health insurance.
- Staged Accidents: Some people intentionally cause accidents or collisions to make fraudulent claims for personal injuries or property damage.
- Identity Theft: Fraudsters may use stolen identities to apply for insurance policies or make claims under someone else’s name.
- Arson: In property insurance, arson is a common form of fraud where policyholders intentionally set fire to their property to claim insurance money.
- Medical Billing Fraud: In health insurance, providers or policyholders may engage in billing fraud by submitting false or inflated medical bills.
- Exaggerated Losses: Policyholders may exaggerate the extent of their losses when filing a claim, seeking a higher payout than justified.
The impact of fraud reverberates through financial, operational, and trust dimensions. Insurance fraud can have several negative impacts on insurance companies, policyholders, and the overall economy. Here are some key consequences:
Financial Losses: Insurance fraud leads to financial losses for insurance companies. The need to pay out fraudulent claims or conduct lengthy investigations can strain the financial resources of insurers.
Increased Premiums: To offset the losses incurred due to fraud, insurance companies may raise premiums for all policyholders. This can result in higher costs for honest customers who end up paying more to cover the losses caused by fraudulent activities.
Reduced Trust: Fraud erodes trust between insurers and policyholders. When insurance companies have to scrutinize claims more thoroughly, it can create an atmosphere of suspicion and mistrust, affecting the overall relationship.
Resource Drain: Investigating and combating fraud requires significant resources, including time and money. Insurance companies need to invest in advanced technologies and skilled personnel to detect and prevent fraudulent activities.
Legal Consequences: Insurance fraud is a criminal offense, and those caught engaging in fraudulent activities may face legal consequences, including fines and imprisonment. This can tarnish the reputation of both individuals and the insurance industry as a whole.
Market Distortion: Fraudulent activities can distort the insurance market by creating an uneven playing field. Honest insurers may struggle to compete with those who engage in fraud to reduce costs or gain a competitive advantage.
To address these challenges, insurance companies continually enhance their fraud detection mechanisms, collaborate with law enforcement agencies, and invest in technologies to stay ahead of evolving fraudulent tactics.
AI-driven fraud detection utilizes advanced algorithms and machine learning techniques to analyze vast amounts of data in real-time, aiming to identify patterns, anomalies, and suspicious activities indicative of fraudulent behavior within the insurance sector. By leveraging predictive modeling, natural language processing, and image analysis, AI systems can detect irregularities in claims submissions, policyholder behavior, and associated documentation. These systems continuously learn from historical data, adapting and evolving to stay ahead of emerging fraud tactics. Through the automated analysis of complex relationships, network patterns, and deviations from established norms, AI-driven fraud detection not only enhances the accuracy of identifying fraudulent activities but also facilitates quicker response times, ultimately safeguarding insurance companies from financial losses and preserving the trust of honest policyholders.
Combining Robotic Process Automation (RPA) with AI-driven fraud detection can create a synergistic effect, enhancing the efficiency and impact of fraud prevention efforts in the insurance sector. Here’s how you can integrate both technologies:
Data Integration and Extraction
RPA can be used to automate the extraction of relevant data from various sources, such as claim forms, emails, and documents. AI algorithms can then analyze this data for patterns and anomalies to identify potential fraud.
Claim Processing Automation
RPA can automate routine and rule-based tasks in the claims processing workflow, such as data entry and validation. AI can be integrated to analyze the processed data for any irregularities or signs of fraud.
Real-time Monitoring
RPA bots can continuously monitor incoming data and trigger alerts for potential issues. AI algorithms can analyze these alerts in real-time, allowing for immediate action to be taken when fraudulent activities are detected.
Fraudulent Pattern Recognition
RPA can assist in automating the identification and tracking of specific patterns associated with fraudulent activities. AI algorithms can analyze historical and real-time data to refine and update these patterns for more accurate fraud detection.
Automated Communication and Reporting
RPA can handle the automation of communication processes, sending alerts or notifications to relevant stakeholders when potential fraud is detected. AI can provide detailed insights and reports on fraudulent patterns, contributing to strategic decision-making.
Integration with Legacy Systems
RPA can bridge the gap between legacy systems and newer technologies by automating interactions and data transfers. AI can then process and analyze the integrated data for fraud detection.
Efficiency in ClaimsInvestigation
RPA can streamline the initial stages of claims investigation by automating repetitive tasks. AI can provide deeper analysis and insights, helping investigators focus on complex cases that require human judgment.
In the relentless battle against fraud, the marriage of cutting-edge technologies, namely Robotic Process Automation (RPA) and Artificial Intelligence (AI), emerges as a formidable ally. RPA’s prowess in automating routine tasks streamlines processes, reducing the human error quotient and enhancing overall operational efficiency. Meanwhile, the cognitive capabilities of AI, including predictive modeling, pattern recognition, and real-time analysis, elevate fraud detection to unprecedented levels of accuracy and agility. The seamless integration of these technologies not only fortifies the defenses of industries, such as insurance but also underscores a paradigm shift in the approach to combating deceptive practices. As we navigate the intricate landscape of fraud prevention, the symbiotic relationship between RPA and AI stands as a beacon of hope, empowering sectors to stay one step ahead in an ever-evolving world of fraudulent challenges.
Talk to our Experts to learn more