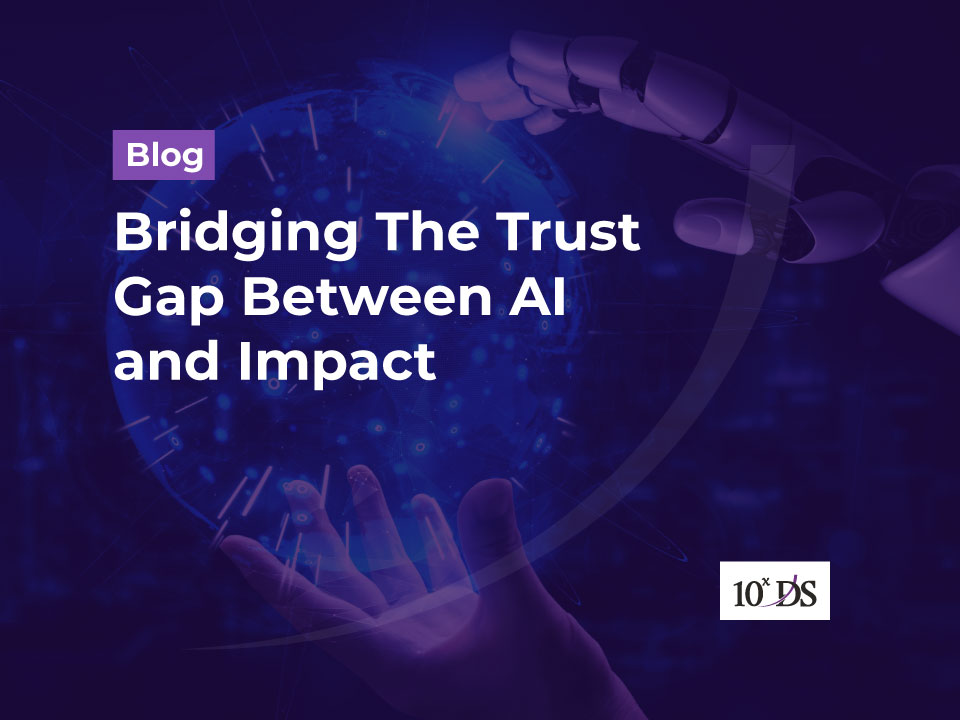
Bridging The Trust Gap Between AI And Impact
Artificial intelligence (AI) technology is quickly becoming a potential disrupter and essential enabler in every industry. The benefit and promise of AI for society and business are undeniable. Artificial Intelligence is helping people make better predictions and informed decisions, enabling innovation and productivity gains. It is used for security purpose, medical assistance and even in facilitating the global fight against COVID-19. But many companies are not taking full advantage of the benefits it has to offer. A major factor standing between AI and impact is a lack of trust. According to 2021 Forrester survey data, data and analytics decision-makers whose firms are adopting AI claim that these issues include privacy concerns with the use of AI to mine customer insights, inability to maintain oversight and governance of machine decisions and actions, and concern about unintended, potentially negative, and unethical outcomes. These issues are causing public concern and raising questions all around the world about the trustworthiness and regulation of AI systems.
AI presents its own trust-related challenges because:
- AI is self-programming, through machine learning.
- AI predictions are not deterministic. Business leaders will need to translate AI’s probabilities into a specific business context.
- AI is a moral mirror where it replicates natural human shortcomings like bias, discrimination, and injustice.
As these incidents continue to make headlines, stakeholder trust in AI will remain shaky. If AI systems do not prove to be worthy of trust, their widespread acceptance and adoption will be hindered, and the potentially vast societal and economic benefits will not be fully realised. Human trust typically comes from a foundation of understanding each other’s motivations, reasoning methods and the likelihood of how each party may react to a variety of situations. This level of understanding comes from a thesis of similarity. With AI, the foundation of similarity is missing. Trust is earned based on track record, transparency and understanding of how the machine will react to new situations. Some essential building blocks of AI trustworthiness include Accuracy, Explainability, Interpretability, Privacy, Reliability, Robustness, Safety, Security, Resilience and Mitigation of harmful bias which we shall see more detailed.
In short, there needs to be an acceptance or conviction that a machine will work as designed and intended. The tasks should be repeatable, predictable, and reliable
Trustworthiness in Technical Point:
Trust comes from knowing an AI system has the following distinct technical properties.
- Accuracy: A system performs designated and predictive tasks with a high degree of accuracy and a low error rate.
- Robustness: Statistically speaking, a system deals well with outliers and environmental changes. It recognizes changes it cannot handle and shuts down gracefully rather than crashing, spewing nonsense or going completely awry.
- Resiliency: As environmental changes occur; a system adapts and learns to recover functionality and a path to thrive in the new conditions.
- Security: A system cannot be hacked or hijacked and resists attacks.
- Explainability: When technical requirements call for human explanation, system actions and decisions are justified in understandable ways.
Trustworthiness in Social and Ethical perception:
In social and ethical contexts AI is often referred to as “responsible AI,” where it characterises competence, expertise and relatable human trust traits like reputation and good governance. Few traits of a responsible AI could be:
- Privacy: A system does not violate user or subject privacy.
- Fairness/ Mitigation of Human bias: The data and algorithms of a system prohibit bias and prevent unfair treatment towards any segment of the population.
- Interpretability: Humans can understand how a system functions, reaches a decision and why it exhibits particular reactions.
- Transparency: People have visibility into a system’s process and its policies.
- Accountability: The system complies with laws and policies. Its functions are traceable, and its actions are accountable.
To make AI trustworthy and keep developments healthy, it should be done in highly applied settings that demonstrate real, credible solutions to actual problems and an ability to cope robustly with live data and environments. Establishing definitions and producing effective training mechanisms for talent development in this field is best done by working in an environment where applications uncover real issues and address company needs.
Governance and Compliance
Trustworthy AI requires governance and regulatory compliance throughout the AI lifecycle from ideation to design, development, deployment, and machine learning operations that are transparent, fair, dependable, respecting privacy, secure, responsible, and accountable. At its foundation, AI governance encompasses all the above stages and is embedded across technology, processes, and employee training. This includes adhering to applicable regulations, as it prompts risk evaluation, control mechanisms, and overall compliance. Together, governance and compliance are the means by which an organization and its stakeholders ensure AI deployments are ethical and can be trusted.
Conclusion
It is the user, the human affected by the AI who ultimately places their trust in the system. No longer are we asking automation to do human tasks, we are asking it to do tasks that we can’t. Moreover, AI has the ability to learn and alter its own programming in ways we don’t easily understand. The AI user has to trust the AI because of its complexity and unpredictability, changing the dynamic between user and system into a relationship. Alongside research toward building trustworthy systems, understanding user trust in AI will be necessary in order to achieve the benefits and minimize the risks of this new technology.
In order to achieve the potential of human and machine collaboration, organizations need to communicate a plan for AI that can be generally adoptable. By having an ethical framework in place, organizations create a common language by which to articulate trust and help ensure integrity of data among all of their internal and external stakeholders. Having a common framework and lens to apply the governance and management of risks associated with AI consistently across the enterprise can enable faster, and more consistent adoption of AI.
Talk to our experts to improve your business operations and generate more value for your company by leveraging AI. Our team can help you grasp the AI methodologies, select the appropriate AI technology adoption and its implementation for your company.