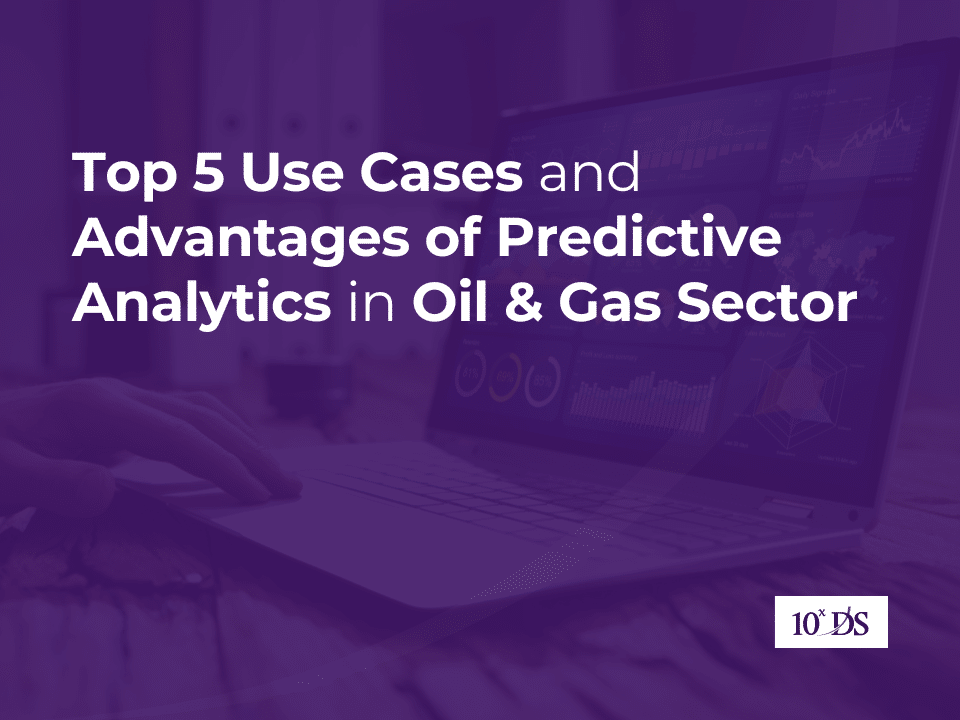
Top 5 Use Cases and Advantages of Predictive Analytics in Oil & Gas Sector
Industries including oil & gas, relied on a variety of traditional methods for decision-making, forecasting, and maintenance. While these methods were effective to an extent, they were typically reactive. Manual data analysis and expert judgment were prone to errors, bias, and inconsistencies. These methods could not handle large, complex datasets or account for multi-dimensional relationships. In many industries, reactive maintenance and inefficient resource allocation resulted in high operational costs and increased safety risks. But the advent of predictive analytics enabled organizations to anticipate future trends, optimize operations, and make proactive decisions based on data. By leveraging historical data, machine learning models, and statistical techniques, companies can identify patterns, assess risks, and forecast future outcomes with a high degree of accuracy.
Importance of Predictive Analytics:
- Enhanced Decision-Making: Predictive models provide insights into future events, allowing companies to make more informed decisions.
- Cost Optimization: By predicting equipment failures or market demand, companies can allocate resources more effectively, minimizing operational costs.
- Risk Management: Identifies potential risks and mitigates them before they materialize, whether in financial sectors (e.g., fraud detection) or supply chain logistics.
- Improved Customer Experience: Predicting customer behavior helps businesses personalize offerings and anticipate customer needs.
- Operational Efficiency: Optimizes workflows, maintenance schedules, and production processes, leading to improved efficiency and reduced downtime.
Importance of Predictive Analytics in the Oil & Gas Industry:
The oil & gas industry is characterized by complex operations, high capital expenditures, and volatile market conditions. Predictive analytics has become an invaluable tool for addressing these challenges. Its applications can be broadly divided into three main areas:
Asset Maintenance & Predictive Maintenance:
Predictive analytics helps foresee equipment failures before they occur by monitoring sensor data, environmental conditions, and historical maintenance logs. This involves gathering real-time data from machinery, such as temperature, pressure, and vibration levels, and using this information to identify patterns that could indicate an impending failure. As a result, predictive maintenance enables companies to schedule repairs proactively, rather than reacting to unexpected breakdowns. This approach reduces costly unplanned downtime and prevents catastrophic failures, extending the lifespan of expensive machinery and improving overall operational efficiency. For example, in the oil & gas industry, predictive models are used to anticipate corrosion in pipelines or detect wear and tear in drilling equipment, allowing for timely intervention.
Production Optimization:
Oil & gas production involves complex geological formations and sophisticated extraction methods that require precise planning and control. Predictive analytics can optimize these processes by analyzing a variety of data inputs, including seismic data, reservoir characteristics, and production history. By doing so, predictive models help determine optimal drilling locations, production rates, and extraction methods, which ultimately maximize output and reduce waste. In upstream operations, these models can be used to simulate different production scenarios and predict well performance under various conditions. A specific example includes using real-time data to adjust fracking techniques, optimize well placements, and fine-tune production settings, leading to enhanced productivity and lower operational costs.
Supply Chain & Market Forecasting:
Predictive analytics can significantly enhance supply chain management and market forecasting in the oil & gas industry. By leveraging advanced algorithms and historical data, companies can accurately forecast oil and gas prices, as well as demand-supply dynamics. This allows for more refined production planning and better alignment of supply with market needs. Additionally, predictive analytics enables optimization of logistics and inventory management, ensuring that supplies are available when and where needed, reducing the risk of overstocking or shortages. For instance, predictive models can anticipate the impact of geopolitical events, natural disasters, or seasonal variations on market demand, helping companies adjust their strategies accordingly and remain agile in volatile market conditions.
Health, Safety, and Environmental (HSE) Monitoring:
The oil & gas industry is inherently high-risk due to hazardous working conditions and the potential for severe environmental impacts. Predictive analytics plays a critical role in enhancing health, safety, and environmental monitoring by analyzing a range of data, including safety records, weather data, and equipment conditions. By identifying patterns and correlations that indicate potential safety hazards, predictive models can help companies implement preventative measures before incidents occur. This includes anticipating environmental risks, such as oil spills or toxic emissions, and taking corrective actions to prevent these occurrences. For example, machine learning models can be used to predict the probability of safety incidents in high-risk areas, enabling companies to focus on risk mitigation and accident prevention.
Exploration & Drilling:
Exploration and drilling are costly and high-risk activities in the oil & gas industry. Predictive analytics can significantly improve exploration outcomes by analyzing geological data to identify new potential reserves more accurately and cost-effectively. By using a combination of geospatial and seismic data, companies can create detailed subsurface maps and predict the locations of oil and gas reserves, reducing the risk of drilling dry wells. Predictive models can also simulate the outcomes of various drilling strategies, allowing companies to select the most efficient and cost-effective approach. For example, advanced machine learning models can interpret 3D seismic data to predict subsurface formations, helping companies target promising drilling sites and optimize resource allocation.
The future of predictive analytics in the oil and gas industry will be defined by the integration of AI, machine learning, and digital technologies like the Internet of Things (IoT) to deliver more real-time, data-driven insights. Companies will increasingly adopt digital twins—virtual replicas of physical assets—to simulate operations and optimize performance. This, coupled with real-time sensor data and advanced AI models, will enable highly accurate predictions, transforming areas such as asset maintenance, production optimization, and safety management. As a result, the industry will see improved operational efficiency, reduced downtime, and enhanced decision-making, positioning predictive analytics as a core driver of digital transformation in the sector.
Talk to our Experts to learn more.