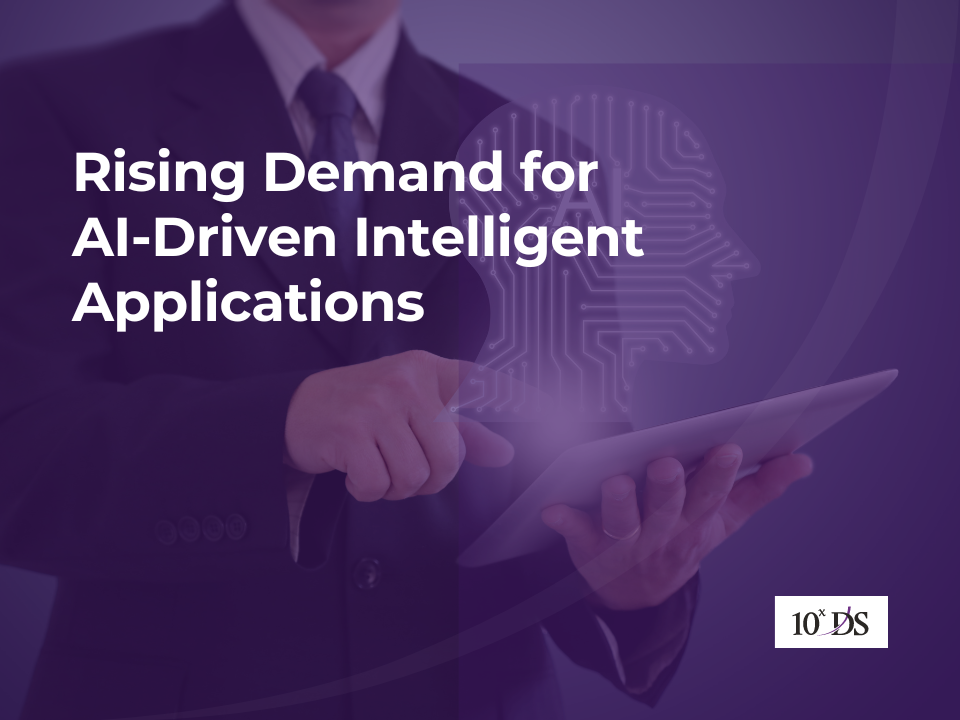
Rising Demand for AI-Driven Intelligent Applications
The evolution of intelligent applications powered by AI has been a journey from theoretical concepts to practical implementations that are now integral to modern life. Advances in machine learning, deep learning, and the availability of vast amounts of data have driven this transformation, leading to sophisticated AI systems that continue to push the boundaries of what is possible. As AI technology evolves, it promises to bring even more profound changes across industries and society as a whole. The 1990s saw a resurgence in AI research with the advent of machine learning, which emphasized learning from data rather than relying on hardcoded rules. Techniques like decision trees, support vector machines, and neural networks gained traction. AI applications in speech recognition, natural language processing, and computer vision started to emerge. The explosion of the internet provided vast amounts of data, fuelling the development of more sophisticated machine learning models.
The 2010s marked significant advancements in AI with the rise of deep learning, a subset of machine learning involving neural networks with many layers. The proliferation of big data and improved computational power, particularly GPUs, enabled the training of deep learning models on massive datasets. AI began to be integrated into everyday applications, such as virtual assistants (Siri, Alexa), recommendation systems (Netflix, Amazon), and autonomous vehicles. AI has become ubiquitous, impacting various sectors including healthcare, finance, retail, manufacturing, and more. Intelligent applications are now capable of performing complex tasks with high accuracy and efficiency. The rise of generative AI models, such as OpenAI’s GPT series and DALL-E, has revolutionized content creation, enabling machines to generate text, images, and other forms of media. The integration of AI with edge computing and IoT devices has enabled real-time processing and decision-making at the edge, reducing latency and improving efficiency.
AI is experiencing exponential growth across various sectors. Here are some key statistics and examples of AI adoption across multiple industries: A survey by McKinsey revealed that 50% of companies have adopted AI in at least one business function. And according to Adobe, 47% of digitally mature organizations have a defined AI strategy. And Grand View Research suggests that the global AI market size is expected to reach USD 190.61 billion by 2025, growing at a CAGR of 36.62% from 2018 to 2025.
If we evaluate the AI adoption in multiple Industries, we can see groundbreaking transformations which completely disrupts the industry and all the stakeholders.
Healthcare:
Diagnostics: AI applications like IBM Watson and Google’s DeepMind are improving diagnostic accuracy and speed.
Treatment Personalization: AI-driven tools help in tailoring personalized treatment plans based on patient data.
Operational Efficiency: AI optimizes hospital operations, predicting patient admissions and automating administrative tasks.
Finance:
Fraud Detection: Financial institutions use AI to detect and prevent fraudulent transactions. For example, JPMorgan Chase uses AI for fraud detection, reducing investigation time by 90%.
Algorithmic Trading: AI algorithms analyze market data to make trading decisions, improving the profitability of investment strategies.
Customer Service: Chatbots and virtual assistants, like those powered by Amelia, handle customer inquiries, providing 24/7 support.
Retail:
Personalized Recommendations: E-commerce platforms like Amazon use AI to recommend products based on user behavior and preferences.
Inventory Management: AI helps retailers optimize inventory, reducing overstock and stockouts through predictive analytics.
Customer Insights: Retailers leverage AI to analyze customer data, enhancing marketing strategies and customer engagement.
Manufacturing:
Predictive Maintenance: AI predicts equipment failures before they occur, minimizing downtime and maintenance costs.
Quality Control: AI-powered vision systems inspect products in real-time, ensuring high-quality standards and reducing defects.
Supply Chain Optimization: AI optimizes supply chain logistics, improving efficiency and reducing costs.
Transportation and Logistics:
Autonomous Vehicles: Companies like Tesla and Waymo are developing AI-driven self-driving cars, enhancing safety and efficiency.
Route Optimization: AI algorithms optimize delivery routes, reducing fuel consumption and improving delivery times.
Fleet Management: AI monitors vehicle health and performance, optimizing fleet operations and maintenance schedules.
Adopting AI-powered intelligent applications requires thoughtful planning and consideration to ensure successful implementation and sustainable growth. Organizations must start by defining clear objectives and identifying specific use cases where AI can provide the most value, focusing on areas with measurable outcomes. High-quality, relevant, and secure data is essential, and robust data governance practices must be in place to comply with privacy regulations. Investing in scalable infrastructure, selecting the right AI tools, and ensuring interoperability with existing systems are critical. Skill development through training and hiring AI experts, alongside fostering collaboration between AI and domain experts, is necessary for successful execution. Ethical considerations and bias mitigation are paramount, requiring fairness, transparency, and regular reviews for compliance. Continuous monitoring, performance tracking, and regular model updates are vital for maintaining accuracy and relevance. Budget planning, ROI analysis, and cost-efficient solutions, such as cloud-based AI services, are important for effective cost management. Collaboration with AI vendors, research institutions, and forming strategic partnerships can enhance expertise and resource access. Regulatory compliance and adherence to industry standards must be ensured, while comprehensive user training and change management strategies will facilitate smooth transitions and user adoption. By addressing these aspects, organizations can harness AI’s full potential, driving innovation, efficiency, and competitive advantage while ensuring sustainable growth in the rapidly evolving AI landscape.
Talk to our experts to learn more.