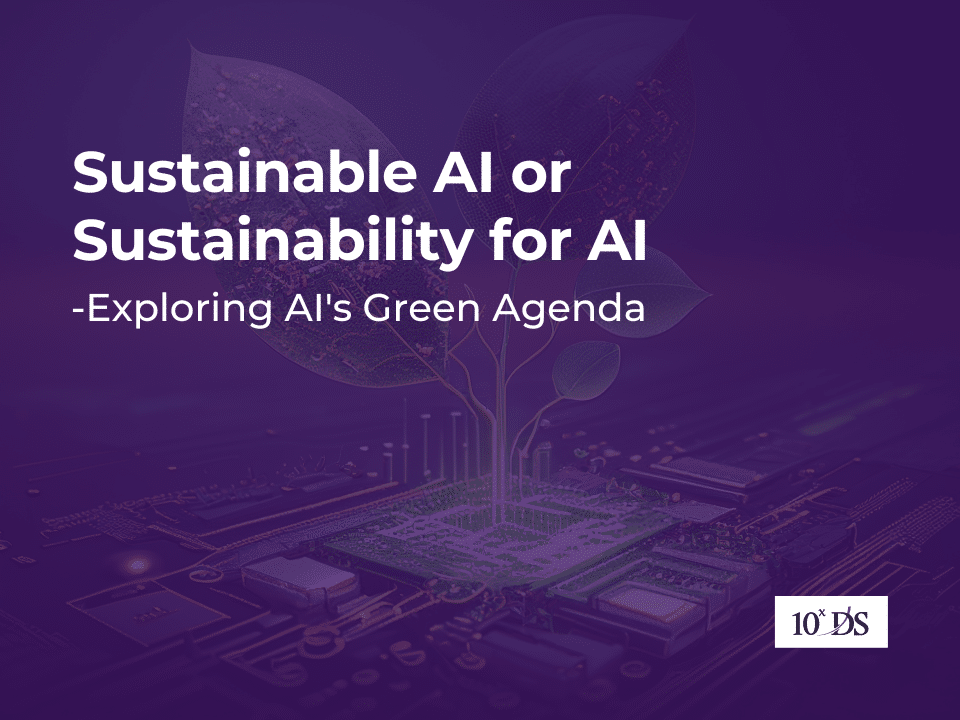
Sustainable AI or Sustainability for AI – Exploring AI’s Green Agenda
As the world grapples with pressing environmental challenges, the role of artificial intelligence (AI) in promoting sustainability has become increasingly prominent. In this blog, we delve into the innovative ways in which AI technology is being harnessed to address environmental concerns and drive sustainable development across various sectors. From energy efficiency and conservation to biodiversity protection and climate resilience, join us as we explore the transformative potential of AI in building a more sustainable future for our planet.
Sustainable AI (Green AI or Eco-friendly AI) focuses on developing and deploying AI technologies in a manner that minimizes their environmental impact and maximizes long-term sustainability. Environmental Dimension aims to reduce the carbon footprint associated with AI systems with strategies include minimizing energy consumption, using sustainable materials in hardware, and reducing electronic waste. For instance, some AI models consume energy equivalent to driving five average American cars over their lifetime during training. Social Dimension (S) ensures that AI respects human rights, promotes fairness, protects user privacy, and contributes positively to society. It addresses challenges related to AI-generated images and ethical practices. It also has a Governance Dimension (G) that aligns AI development with responsible and ethical principles. It Promotes positive environmental, social, and governance outcomes. Developing sustainable AI involves implementing practices and techniques that minimize environmental impact, promote ethical considerations, and ensure long-term viability. Here are several ways to achieve sustainable AI:
Energy Efficiency
Design AI algorithms and hardware to be energy-efficient, reducing the carbon footprint associated with AI computations. For instance, optimizing machine learning models to require fewer computational resources while maintaining performance, or using renewable energy sources to power AI infrastructure.
Data Efficiency
Develop AI systems that require less data to operate effectively. This can involve techniques like transfer learning, where models are pre-trained on large datasets and fine-tuned on smaller, domain-specific datasets, reducing the need for massive data collection and storage.
Algorithmic Fairness and Transparency
Ensure AI systems are fair and transparent, avoiding biases and discriminatory outcomes. For example, implementing fairness metrics during model training to detect and mitigate biases, or providing explanations for AI decisions to increase transparency and accountability.
Recycling and Sustainable Materials
Utilize sustainable materials and practices in manufacturing AI hardware. This includes using recycled materials for hardware components, designing for disassembly and recycling at the end of the product lifecycle, and minimizing electronic waste.
Reducing Digital Divide
Address the digital divide to ensure equitable access to AI technologies worldwide. This involves initiatives such as providing affordable internet access, promoting digital literacy programs, and developing AI solutions tailored to the needs of underserved communities.
Smart Grids and Energy Management
Implement AI-driven smart grid technologies for efficient energy distribution and management. This involves using AI algorithms to optimize energy consumption, predict demand, and integrate renewable energy sources into the grid effectively, thus reducing reliance on fossil fuels and mitigating greenhouse gas emissions.
By integrating these principles and examples into AI development practices, we can work towards creating sustainable AI systems that not only deliver value but also contribute positively to environmental and societal well-being.
AI for Sustainability refers to using machine learning systems to mitigate the adverse effects of climate change and other environmental challenges. It focuses primarily on addressing environmental issues. Leverages AI to create solutions for sustainability, such as climate modeling, resource optimization, and conservation efforts.
Biodiversity Monitoring with AI
Organizations such as Stream Ocean, formerly known as the Nature Counts Foundation, utilize AI and machine learning to conduct real-time monitoring of marine biodiversity using underwater video cameras. This innovative technology plays a crucial role in coral restoration initiatives by offering sophisticated ocean data analytics, which includes valuable biodiversity metrics essential for the success of such projects.
Climate Resilience with AI
Pano AI harnesses the power of artificial intelligence to detect, verify, and classify wildfire events in real time. This proactive approach significantly contributes to enhancing global resilience against climate-related disasters stemming from forest fires, thus playing a pivotal role in promoting sustainable disaster management strategies.
Energy Efficiency and Pollution Reduction
AI-optimized models present innovative strategies for biodiversity protection while concurrently reducing energy consumption, mitigating electricity waste, and tackling issues related to water pollution and scarcity. Furthermore, the integration of AI holds promise in the development of environmentally sustainable transportation networks, underscoring its multifaceted potential in fostering ecological balance and resilience.
Environmental Monitoring
Leverage AI, particularly natural language processing (NLP), for environmental monitoring and conservation efforts. For example, analyzing social media data to detect and respond to environmental concerns, or using NLP to process and extract insights from large volumes of scientific literature related to climate change.
Precision Agriculture
Apply AI technologies like machine learning and IoT sensors in precision agriculture to optimize resource use, reduce environmental impact, and increase crop yields. This includes using AI to analyze data on soil health, weather patterns, and crop growth to inform targeted interventions such as precision irrigation and pest management.
Both fields hold promise in reshaping industries and creating a greener, more harmonious, and socially responsible world. By embracing energy-efficient algorithms, promoting data transparency and fairness, and leveraging AI-driven solutions in areas such as renewable energy, conservation, and smart resource management, we have the opportunity to drive positive change and build a more resilient, equitable, and sustainable future. However, it is imperative that we remain vigilant in addressing ethical concerns, mitigating unintended consequences, and ensuring that AI technologies are deployed in ways that prioritize environmental stewardship and societal well-being. Together, let us continue to harness the power of AI innovation to advance sustainability goals and safeguard the planet for generations to come.