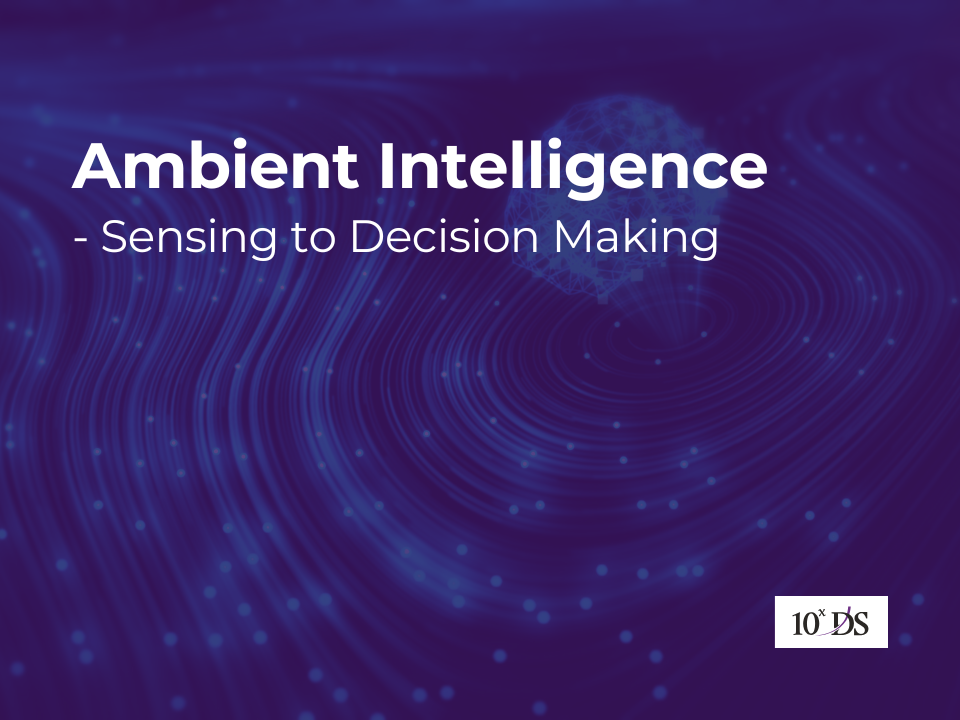
Ambient Intelligence – Sensing to Decision Making
Clothes with embedded sensors that communicate with washing machines to ensure proper care — sounds like wild imagination, doesn’t it? It is not an imagination anymore. Making use of ambient technology and artificial intelligence, Ambient Intelligence is steadily moving from concept to real-life applications, and we’re already seeing it pop up in ways that are subtle but impactful.
Starting from simple sensors designed for tracking and sensing, ambient intelligence has evolved into systems capable of making context-aware decisions and adapting autonomously to human needs. Early implementations focused on collecting data—like motion detectors or temperature sensors—but over time, advances in artificial intelligence and machine learning have enabled these systems to interpret information, learn from patterns, and act proactively. Today, ambient intelligence is not just about sensing the environment, but understanding it deeply enough to offer personalized, real-time support across various domains. Whether it’s adjusting home lighting based on mood, optimizing patient care in hospitals, or streamlining workflows in smart offices, Ambient Intelligence has grown from passive observation to intelligent intervention, quietly transforming the spaces we live and work in. Three major technologies driving this trend are:
Ultra-low-power wireless communication: Bluetooth is currently the most widely used, but other options like Wi-Fi, 5G, and backscatter communication could become more prominent in the future.
Energy harvesting: This allows tiny, battery-free devices to operate indefinitely by drawing power from their surroundings.
Affordable, energy-efficient electronics: These make it possible for compact chips to function using harvested energy, powering sensors and transmitting basic wireless signals.
According to Gartner, through 2028, early examples of ambient intelligence will focus on reducing costs and improving efficiencies via low-cost item tracking and sensing. Some early examples are:
- Fashion retailers using smart fitting rooms with RFID tags to track which items are being tried on, how often, and in what combinations — helping with inventory decisions and product placement.
- Big-box stores using shelf-level sensors to detect low stock or misplaced items, enabling automatic restocking alerts or staff notifications to prevent out-of-stock situations.
- Convenience stores leveraging smart cameras and weight sensors to enable checkout-free experiences (à la Amazon Go), tracking what customers pick up and automatically billing them.
- Cold chain logistics companies using real-time temperature and humidity monitoring during transportation to ensure food, vaccines, or biologics stay within safe ranges — with alerts if conditions deviate.
- Pharmacies integrating intelligent dispensers that log when medications are removed, ensuring compliance and helping track inventory automatically.
- Quick-service restaurants using IoT-connected kitchen equipment to monitor cooking times, food freshness, and equipment maintenance needs to reduce downtime and waste.
- Warehouses and fulfillment centers deploying robots and AI to map item locations in real time and optimize order picking routes for faster delivery.
Getting Ambience Intelligence ready
The process of getting ambient intelligence technology ready starts by clearly defining the problem it’s meant to solve. For instance, let’s say a grocery retailer wants to reduce food spoilage. The first step is identifying where and how the spoilage occurs — maybe in refrigerated sections due to unnoticed temperature fluctuations. The retailer would then choose appropriate sensors (like temperature and humidity sensors) and a wireless communication method (e.g., Bluetooth or Wi-Fi) to continuously monitor these conditions. Next, software is developed to collect this data and apply AI algorithms that detect when temperatures go out of range and automatically send alerts to staff or even trigger cooling system adjustments. This system would be tested in one or two stores to ensure accuracy and reliability, and once proven, it could be scaled chain-wide. Throughout, the team ensures the solution integrates with the retailer’s existing systems and follows privacy and data protection standards. The result is a smart, automated environment that prevents spoilage before it happens—saving money and reducing waste.
Implementing Ambience Intelligence
Implementing ambient intelligence systems is a strategic process that requires thoughtful planning, the right mix of technologies, and a clear understanding of the environment in which the solution will operate. Because Ambience Intelligence involves integrating sensors, connectivity, data analytics, and automation into everyday spaces, the process must balance technical feasibility with user experience and business goals. Whether it’s for a retail store tracking inventory in real time or a healthcare facility monitoring patient environments, successful deployment relies on a structured, step-by-step approach. Below are the key stages involved in bringing ambient intelligence from concept to reality.
1. Requirement Analysis & Use Case Definition
The first stage involves deeply understanding the problem you’re trying to solve or the opportunity ambient intelligence can unlock. This includes identifying the environment where the system will operate (e.g., retail store, warehouse, hospital) and defining the specific goals—such as reducing inventory loss, enhancing energy efficiency, or improving customer experience. Stakeholders collaborate to outline clear, measurable outcomes and prioritize features that the system must deliver. At this point, it’s also important to assess the feasibility of the implementation in terms of cost, scale, and integration with existing workflows.
2. System Design & Planning
Once the use case is clear, the next step is designing how the system will function. This includes selecting the types of sensors needed (e.g., RFID for tracking, temperature sensors for cold chain monitoring), choosing communication methods (Bluetooth, Wi-Fi, 5G), and determining the architecture for data processing and storage. Designers and engineers map out how data will flow through the system—from sensing to analysis to action—and create models for how the system will interact with users or trigger responses. This planning phase also involves setting up requirements for data privacy, security, and system reliability.
3. Technology Selection & Procurement
In this stage, the actual technologies are chosen and procured. This involves selecting vendors for hardware such as sensors, edge devices, and gateways, as well as software platforms for data analytics and artificial intelligence. The focus is on choosing components that are reliable, scalable, and compatible with each other and with the existing IT infrastructure. Cost-effectiveness, power efficiency (especially for battery-free or energy-harvesting devices), and ease of deployment are also key considerations. Pilot units or development kits may be acquired to begin initial testing.
4. Integration & Development
Now the system components are brought together and integrated into the target environment. Developers build or configure the software to gather, process, and analyze the data coming from the sensors. This might include machine learning models, automation logic, and dashboards or mobile interfaces for users. Integration with existing enterprise systems—like inventory databases, CRM tools, or building management systems—is also addressed here. At this point, it’s crucial to ensure seamless communication across devices and consistent performance across different conditions.
5. Testing, Calibration & Validation
Before full deployment, the system undergoes extensive testing in real-world conditions. This includes verifying that sensors accurately collect data, wireless communication is stable, and software correctly interprets and responds to events. Calibration ensures that thresholds and triggers (like temperature limits or motion detection sensitivity) are appropriate for the environment. User feedback is often gathered during this phase to fine-tune interfaces and responses. Validation confirms that the system meets the original objectives and complies with relevant regulations or industry standards.
6. Deployment & Scaling
Once the system has been validated, it can be deployed at scale. This could mean rolling it out across multiple stores, departments, or locations. Deployment includes setting up new hardware, onboarding users, and providing training where necessary. At this stage, monitoring tools are often implemented to track performance, flag anomalies, and support ongoing maintenance. Scaling may also involve refining algorithms with more data, adjusting configurations for different locations, and updating the system based on evolving business needs.
Privacy concerns around Ambience Intelligence
As ambient intelligence becomes more deeply embedded in everyday objects and environments, privacy quickly becomes one of the biggest challenges. Tracking sensors in clothing, wearables, or personal devices could easily cross the line into tracking individuals — even unintentionally. Here’s how this concern can be addressed:
1. Data Anonymization & Minimization
Systems should be designed to collect only the data that’s absolutely necessary for the intended function, and wherever possible, data should be anonymized. For instance, a sensor in a jacket can signal it’s near a washing machine, but it doesn’t need to know who is wearing it.
2. Clear Consent Mechanisms
Users should always be informed when data is being collected, what it’s used for, and given control over consent. This might involve opt-in features, app notifications, or smart labels that indicate whether an item has embedded sensors.
3. Local Processing (Edge AI)
Processing data locally on devices rather than sending everything to the cloud (known as edge computing) helps reduce privacy risks. For example, a sensor in clothing could process basic info (like wash instructions) right on the chip, without ever transmitting personal data externally.
4. Secure Communication & Storage
All data transmissions should be encrypted, and storage should follow strong security standards (like end-to-end encryption, role-based access, etc.) to prevent unauthorized access or leaks.
5. Regulatory Compliance & Transparency
Complying with regulations like GDPR, CCPA, or any local data protection laws ensures that user rights are protected. It also builds trust when companies are transparent about how and why data is collected and used.
6. Physical Deactivation or Control Options
Give users the ability to disable or remove sensors, especially in personal items. Some smart tags today are designed to deactivate after purchase or after a specific function is complete (e.g., a tag that deactivates after a wash cycle).
Conclusion
The future of ambient intelligence envisions environments seamlessly integrating advanced technologies—such as artificial intelligence (AI), the Internet of Things (IoT), and ubiquitous computing—to create spaces that are responsive, adaptive, and personalized to individual needs. In healthcare, for example, ambient intelligence is poised to revolutionize patient care by enabling devices to monitor health metrics continuously and provide proactive interventions. In smart cities, ambient intelligence can enhance urban living by optimizing traffic flow, reducing energy consumption, and improving public safety through interconnected sensors and real-time data analysis. As AI algorithms become more sophisticated, ambient intelligence will further enhance convenience, efficiency, and personalization, minimizing the need for manual input. However, as these technologies become more pervasive, addressing privacy and security concerns will be paramount to ensure user trust and widespread adoption.
Talk to our experts to learn more!